Dr. Suresh Bhavnani, a biomedical informatics researcher, was used to dealing with detailed medical data and spreadsheets full of numbers and complex statistics. However, when his mother fractured her hip, the anonymous numbers suddenly became personal.
For an older patient, to be readmitted to the hospital within 30 days after a hip fracture surgery can mean an increased risk of infection or death, as well as increased costs for patients and hospitals. Knowing which patient subgroups are at a higher risk of readmission and the real-world processes leading to readmission could help doctors and nurses design better recovery treatment plans. However, current models of risk assessment are not designed to provide such insights, said Bhavnani, a professor
at The University of
Texas Medical Branch at Galveston.
A new paper published in JMIR Medical Informatics by Bhavnani and a team of health care experts at UTMB show how a combination of certain pre-existing conditions could make
an otherwise healthy person become a higher risk patient needing specialized care.
As his mother was generally healthy with well-controlled pre-existing conditions, Bhavnani did not think she would have any problems with her hip surgery, and she did not. The problems started after the operation while she was at a rehab facility.
When his mother ended up back in the hospital for nonsurgical reasons, he was concerned. When she was discharged and readmitted two more times, Bhavnani knew it was time to figure out what was going wrong.
“It turns out that my mom’s readmission experience is not unique,” Bhavnani said.
With funding from the Patient-Centered Outcomes Research Institute, the Sealy Center on Aging, and the Institute for Translational Sciences, Bhavnani and a multidisciplinary team consisting of physicians, rehabilitation specialists, nurses, and data scientists looked at his mother’s case as well as data from more than 33,000 other patients with hip fractures. They found that models designed to predict hospital readmission and the risk assessment of individual patients were not identifying groups of patients who needed extra care.
Through an integrated analysis using Bhavnani’s visual analytical method, the team was able to identify subgroups of patients with hip fractures who shared pre-existing conditions such as renal failure, diabetes, arrhythmia, and others, and map
out how combinations of these conditions could lead to a higher risk of readmissions.
“A hip fracture patient with renal failure or diabetes has a certain risk for readmission but if you combine that with another condition such as congestive heart failure, stroke, COPD, the risk jumps up,” Bhavnani said.
Bhavnani said he observed this firsthand when his mother was at the rehab unit, which focused mainly on making sure her surgical wound was healing and getting her back on her feet through physical therapy. However, due to the new surroundings, medications,
and unfamiliar diet, her prior conditions were exacerbated, landing her back in the hospital, Bhavnani said.
By knowing which high-risk pre-existing conditions interact and affect a patient’s risk of readmission, health care providers could be better prepared and better treat their patients after a hip fracture surgery, he said.
The research team was also able to come up with practical solutions to a thorny problem.
The team’s paper describes how discharge notes and order sets could be designed to alert a physician if a patient had pre-existing conditions that would make a patient have a higher risk and be in need of specialized care, and make that information more prominent to care providers in the rehab unit.
“Bhavnani’s visual analytics model made it immediately clear which preexisting conditions were interacting to increase the risk of readmission in patient subgroups,” said Dr. Mukaila Raji, director of the Division of Geriatrics at UTMB.
“It helped us to put the pieces together into a practical translation that can dramatically reduce the risk of readmission.”
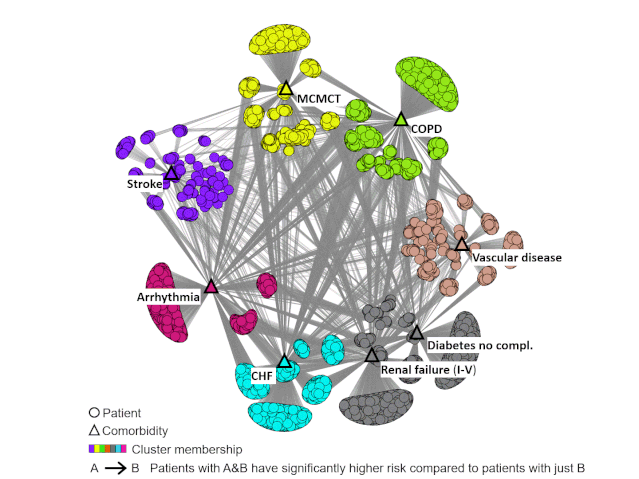
Bhavnani is now using similar approaches to analyze how pre-existing conditions interact to increase the risk of poor outcomes in older patients with COVID-19.
Dr. Bhavnani is professor of biomedical informatics in the Department of Preventive Medicine and Population Health, senior fellow at the Sealy Center on Aging, member of the Institute for Translational Sciences, adjunct faculty at the School of Biomedical
Informatics in UT Houston, and PI of the Discovery and Innovations through Visual Analytics Laboratory (DIVA Lab): http://www.skbhavnani.com/DIVA/contact.html